Bias in Conversational AI Systems: Evaluating and Monitoring LLM for More Accessibility
Nov 21, 2024
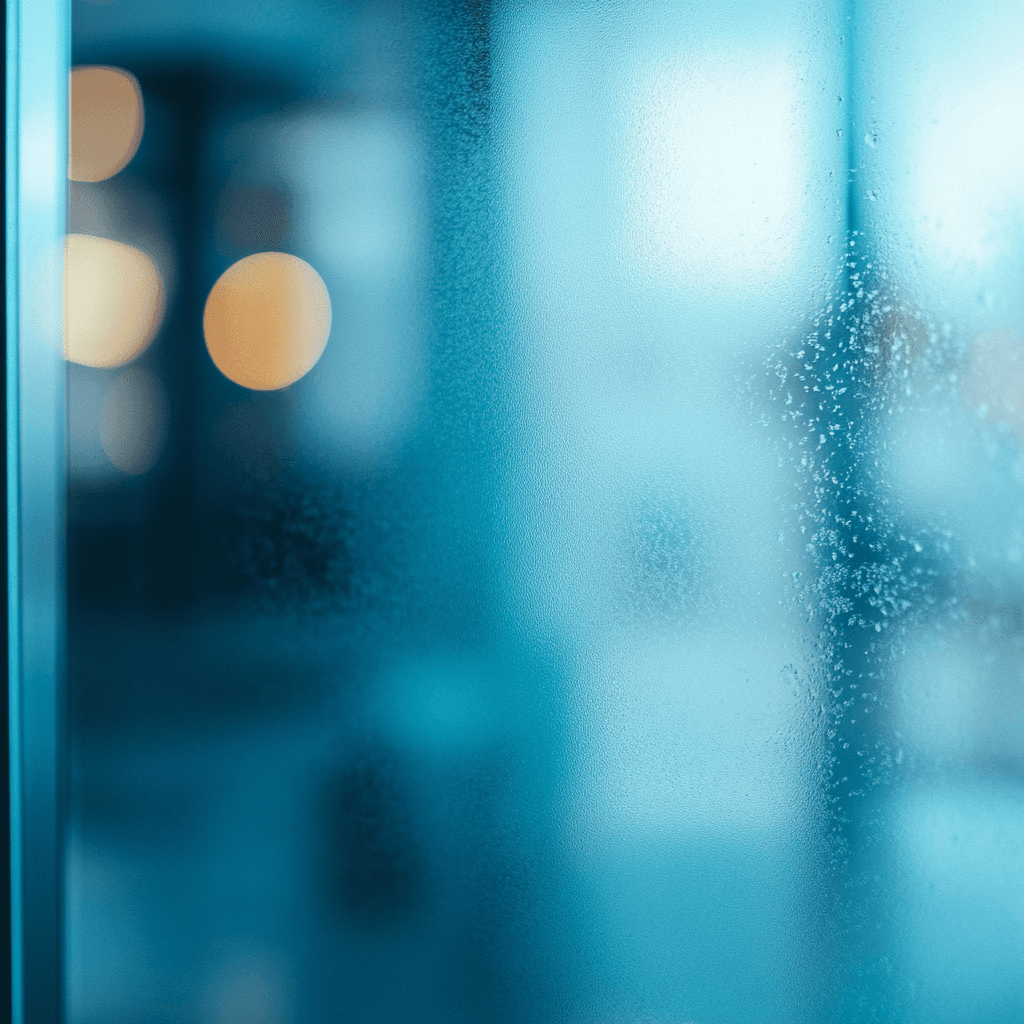
Conversational AI systems, chatbots, virtual assistants and other AI workflows are becoming our everyday companions. Whether we're asking for weather updates or troubleshooting tech issues, these AI systems are becoming the frontline of user interaction with many businesses. But with great power comes great responsibility, and one of the biggest challenges we face is ensuring these systems are free from bias.
What's Causing Bias in Conversational AI Systems?
Bias doesn't just appear out of thin air; it's often a reflection of systemic issues and oversights in design and implementation. Here are some key contributors:
Training Data: If the data fed into the AI is biased, the AI will mirror those biases.
Language and Representation: Nuances in language and cultural expressions can be misinterpreted, leading to misrepresentation of certain groups.
System Design: Choices in user interface, algorithms and frameworks can inadvertently introduce bias.
Accessibility: Failing to consider users with disabilities, including things like dyslexia, can make AI interactions hard to handle.
Cultural and Historical Bias: Existing societal biases can seep into AI systems if not actively mitigated.
Regulatory Oversight: A lack of guidelines and ethical standards can allow biased systems to go unchecked.
The Impact of Bias
Biased AI systems can cause real harm, to users as well as the company releasing the technology. They can marginalise or exclude parts of your user group, and even causing psychological distress. This erodes trust and in the worst case leads to legal repercussions for companies.
Identifying Bias in Conversational AI
So, how do we spot bias in these systems?
Model Evaluations: Before launching, rigorously test AI models and prompts, especially for specific user groups.
Collaborations: Work with organizations to detect misuse and share best practices.
Hazard Taxonomies: Utilize frameworks like the MLCommons® AI Safety benchmark, which assesses models across various hazard categories like hate speech, defamation, and privacy violations.
Evaluating Models: The First Line of Defense
Effective evaluation methods include:
Red Teaming: Actively searching for vulnerabilities by simulating attacks or misuse.
Systematic Evals: Regularly assessing AI responses in controlled scenarios.
Metrics and Benchmarks: Developing and using standardized metrics to measure bias levels.
Continuous Monitoring: Staying Vigilant Post-Launch
Launching an AI system isn't the end—it's the beginning of an ongoing process:
Pattern Detection: Use analytics to spot biased patterns in interactions.
Guard Models: Implement secondary models that monitor the primary AI for biased outputs.
Feedback Mechanisms: Encourage user reports and act promptly on feedback.
Evaluation Loops: Regularly update and reevaluate models with new data.
Actionable Steps Forward
Education: Inform both users and development teams about AI biases and how to address them.
Red Teaming and Monitoring: Make these practices standard in your development cycle.
Collaboration: Engage with industry groups and share insights publicly to build better standards.
Bias in conversational AI is a complex issue, but not one we can't solve. By focusing on thorough evaluation and continuous monitoring, we can create AI systems that are not only intelligent but also fair and inclusive. Let's work together to build a future where AI serves everyone equally.
Ready to transform your AI governance strategy? Visit requesty.ai to explore our solutions.